일 | 월 | 화 | 수 | 목 | 금 | 토 |
---|---|---|---|---|---|---|
1 | 2 | |||||
3 | 4 | 5 | 6 | 7 | 8 | 9 |
10 | 11 | 12 | 13 | 14 | 15 | 16 |
17 | 18 | 19 | 20 | 21 | 22 | 23 |
24 | 25 | 26 | 27 | 28 | 29 | 30 |
- 기초통계
- 히트맵
- Chat GPT
- SQL
- GA4
- 크롤링
- 클러스터링
- cross join
- 데이터 분석
- 머신러닝
- 태블로
- SQLD
- jd
- 서브쿼리
- 팀프로젝트
- 데이터분석
- 전처리
- Python
- da
- 프롬프트 엔지니어링
- If
- 프로젝트
- pandas
- data analyst
- 군집화
- 최종 프로젝트
- 시각화
- lambda
- streamlit
- 기초프로젝트
- Today
- Total
목록데이터 분석 공부 (325)
세조목
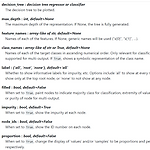
목차 의사결정나무 랜덤 포레스트 KNN(최근접 이웃) 부스팅 알고리즘 1. 의사결정나무 import pandas as pd import seaborn as sns import matplotlib.pyplot as plt from sklearn.preprocessing import LabelEncoder from sklearn.tree import DecisionTreeClassifier, plot_tree titanic_df = pd.read_csv('경로.csv') 의사결정나무를 만들려면 sklearn.tree 라이브러리의 DecisionTreeClassifier 클래스를 가져와야한다. X_features = ['Pclass', 'Sex', 'Age', 'Fare', 'Embarked'] # 평균으로 ..
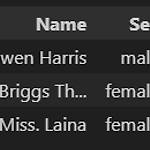
Step 데이터 불러와서 살펴보기 Sibsp(자녀수) + Parch(부모수) 이상치(Outlier) 처리 결측치 처리 인코딩(수치형 데이터) 스케일링(범주형 데이터) 로지스틱회귀(Logistic Regression) / 모델 평가 test 데이터에 적용 1. 데이터 불러와서 살펴보기 train_df = pd.read_csv('경로/train.csv') test_df = pd.read_csv('경로/test.csv') train_df.head(3) train_df.info() train_df.describe(include='all') 다양한 컬럼들이 존재하는데 이 중 'Age', 'Fare', 'Family', 'Embarked', 'Pcalss', 'Sex'를 독립변수로 'Survived'를 종속변수로 ..
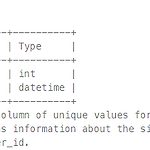
https://leetcode.com/problems/confirmation-rate/description/ LeetCode - The World's Leading Online Programming Learning Platform Level up your coding skills and quickly land a job. This is the best place to expand your knowledge and get prepared for your next interview. leetcode.com Signups와 Confirmations 두 테이블이 있다. 두 테이블을 가지고서 각 유저별 confirm받은 비율을 구하는 것이 문제의 요구사항이다. confirm받을 비율을 구하기 위해서는 각 유저별로..
머신러닝 2024.02.01 - [데이터 분석 공부/머신러닝] - 머신러닝 - 전처리(인코딩, 스케일링)(24.02.01) 머신러닝 - 전처리(인코딩, 스케일링)(24.02.01) 전처리(인코딩 & 스케일링) 인코딩이란 모델이 처리하기 쉬운 값으로 기존 값을 바꾸는 것을 의미한다. 범주형 데이터(Encoding) 레이블 인코딩(Label Encoding) One-Hot Encoding 수치형 데이터(Scaling) 표준 eyeoftheworld1209.tistory.com 2024.02.01 - [데이터 분석 공부/머신러닝] - 머신러닝 - 데이터 분리(feat. 과적합)(24.02.01) 머신러닝 - 데이터 분리(feat. 과적합)(24.02.01) 1. 과(대)적합 과대적합이란 데이터를 너무 과도하게..